Briefing
- Organizations are rapidly adopting AI for competitive advantage, requiring security teams to adapt quickly.
- Security teams must enable safe AI innovation through effective governance and controls.
- Autonomous AI agents are emerging as a new security challenge, requiring updated defense strategies.
- AI tools and models from third parties pose supply chain risks that need thorough evaluation.
- Current regulations need refinement to address specific AI use cases while maintaining security.
- CISOs must lead AI strategy by building organizational AI literacy and addressing security risks.
AI is reshaping how organizations operate, bringing new security challenges for CISOs. Autonomous AI agents, complex vendor relationships, and evolving regulations require updated security approaches.
The “Addressing the AI Readiness Gap” panel at AI House in Davos gathered insights from industry leaders Jeetu Patel (Cisco Systems), Arvind Jain (Glean), Navrina Singh (Credo AI), and Martin Lund (Cisco).
This article presents key findings and offers practical steps for CISOs to secure AI systems while enabling innovation.
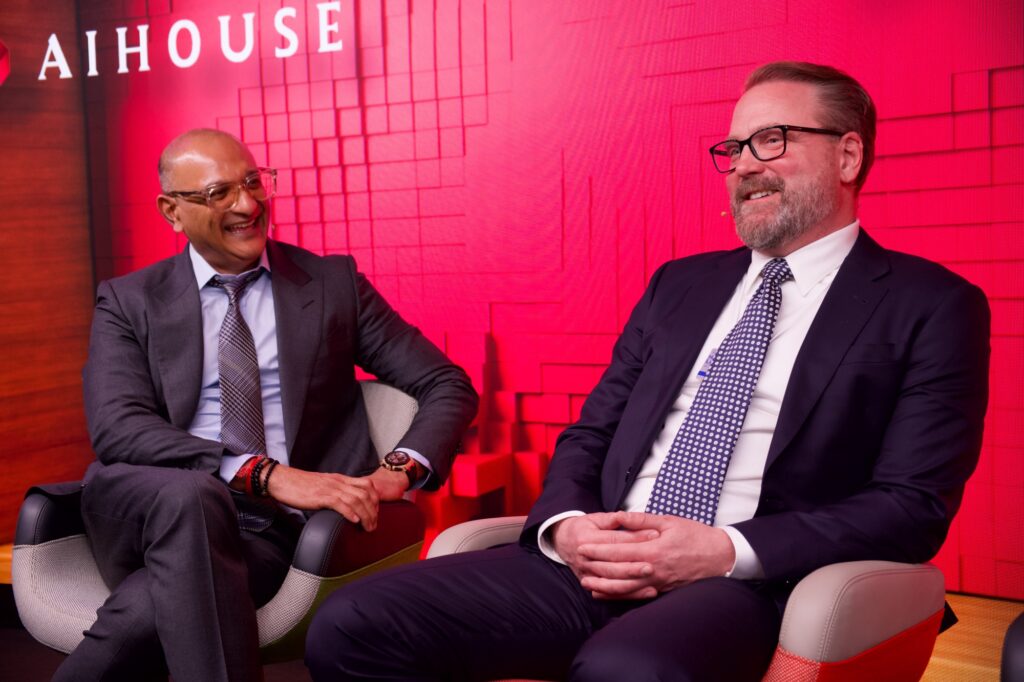
01: AI's Acceleration: Opportunities and Risks
AI is now central to business operations, bringing new security risks. At Davos, industry leaders discussed how this widespread adoption of AI is changing the security landscape.
Key Insights from the Session
Cross-Industry AI Adoption. Arvind Jain from Glean noted that AI use has expanded beyond tech companies. Organizations in every sector use AI to improve operations and compete more effectively.
Accelerated Innovation. Martin Lund, Executive Vice President at Cisco, pointed out that AI development in hardware, software, and models is moving faster than previous technology shifts. This rapid evolution creates new security challenges.
Business Impact. Companies are using AI not just to automate tasks but to create new products and services. As Jain explained, AI capabilities become a key differentiator in the marketplace.
Cyber Implications
Larger Attack Surface. AI systems add new entry points that attackers can target. Each AI deployment – whether using commercial models, custom solutions, or hybrid approaches – creates vulnerabilities beyond traditional IT systems. These include model API exposures, training pipeline weaknesses, and inference endpoint risks. Security teams now face both standard cyber threats and AI-specific attacks like model theft, data poisoning, and prompt manipulation. This security challenge will grow as organizations expand their AI capabilities – Deloitte’s recent survey shows strong interest in emerging AI innovations, with 52% of organizations focusing on agentic AI, 45% on multiagent systems, and 44% on multimodal capabilities.
Rapid Changes. AI systems evolve faster than traditional security controls can adapt. Martin Lund noted that AI technology is advancing 10,000 times faster than previous technology shifts. This speed creates security gaps. Models update frequently, frameworks change monthly, and new attack methods emerge weekly. Standard security practices like quarterly assessments or annual audits fall short. Teams need real-time monitoring and rapid response capabilities to protect AI systems that change daily. Security controls must evolve as quickly as the AI systems they protect. This challenge will intensify – Deloitte’s recent survey shows that 78% of organizations plan to increase their AI spending in the next fiscal year, accelerating the pace of AI adoption and change.
System Complexity. Today’s AI systems combine multiple parts that are hard to secure. Organizations typically use several models from different vendors, run workloads across multiple clouds, and connect various AI tools. This creates a complex network of components to protect. Each part needs specific security controls, yet they all interact in ways that can create unexpected vulnerabilities. The non-predictable nature of AI models makes security monitoring harder – normal behavior is difficult to define, and breaches are harder to spot. Security teams must track the entire AI supply chain, from model sources to deployment environments while ensuring consistent security across all components.
Key Recommendations for CISOs
Build Agile AI Security Programs. Create security frameworks that match AI’s rapid development pace. Update security checks to happen alongside AI development, not after. Establish iterative security assessment approaches integrated with the development lifecycle. Implement rapid patching or remediation of vulnerabilities in AI-related software and frameworks. This shift from traditional security to AI-ready practices helps organizations deploy AI faster while maintaining safety.
Make Security Part of AI Design. Involve security teams from the start of AI projects. Identify potential risks at the design stage. Assess each AI use case for specific threats. Check data handling practices against security requirements. The panel stressed how early security involvement speeds up AI adoption – one financial services company cut their AI testing time from six months to three weeks by implementing proper security controls early.
Deploy Runtime AI Security Controls. Pre-deployment testing alone cannot catch all vulnerabilities in rapidly evolving AI systems. You need active protection while systems are running. Deploy GenAI Shields and LLM firewalls as your first line of defense. These tools monitor AI systems in real time to detect prompt injection attacks, sensitive data leaks, and unusual model behavior. They track unexpected outputs, abnormal response patterns, and suspicious resource usage that could signal security issues. Set up automated responses to block malicious requests before they reach your models. This creates an effective security layer that protects AI operations without impacting development speed.
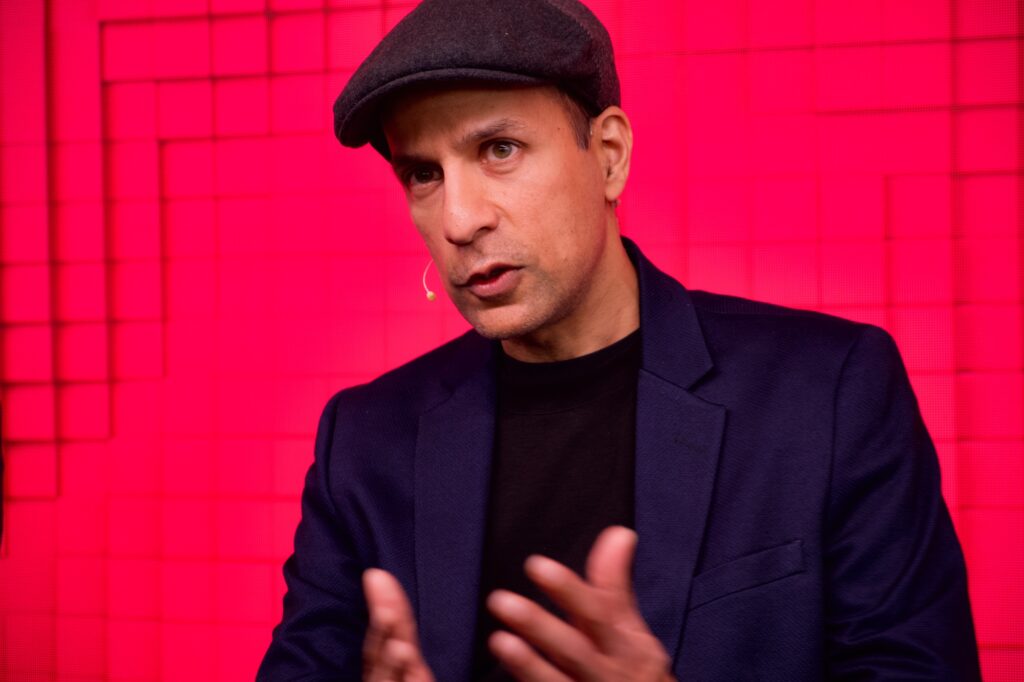
02: Security Enables AI Innovation
Many see security as slowing down AI projects. The panel showed the opposite: good security speeds up AI adoption and helps build better AI systems.
Key Insights from the Session
Security Builds Trust. Narvina Singh shared how proper security controls help companies deploy AI faster. One financial services client cut their AI testing time from six months to three weeks by implementing security controls early. These controls helped prove to their banking customers that their AI systems were safe and reliable.
Prevent Project Delays. Jeetu Patel explained how security problems can entirely stop AI projects. When companies skip security, they often face breaches or compliance issues that force them to pause development. Adding security measures from the start prevents these costly delays. A breach discovered late in development can set projects back months and damage a company’s reputation.
Start Security Early. Arvind Jain noted that companies move faster with AI when security teams join early. His experience shows enterprises struggle to launch AI tools when security reviews happen too late. Security teams can spot risks early, suggest fixes, and help choose the right AI tools. This prevents the common problem of rebuilding AI systems after security issues surface.
The panel stressed a key point: security is not extra work that slows things down. Instead, it helps companies build AI systems right the first time, avoid rework, and launch faster. Companies that treat security as essential rather than optional see better results with their AI projects.
Cyber Implications
Security as a Driver of AI Adoption. By ensuring that AI systems are secure and trustworthy, CISOs can facilitate their adoption across the organization. Strong security practices build confidence among stakeholders, enabling faster and more widespread deployment of AI solutions.
Security Drives Business Growth. Strong AI security practices give organizations an advantage in the market. When companies prove their AI systems are secure and reliable, they build trust with customers and partners. This advantage matters most in industries where trust and data protection are critical.
Security Enables Innovation. Effective risk management creates a secure foundation for AI experimentation. When organizations identify and address security risks early, teams can explore new AI opportunities while maintaining security and compliance standards.
Security Delays Cost More. Fixing security issues late in AI development can halt entire projects. Late-stage security problems often force companies to rebuild systems and damage their market reputation.
Key Recommendations for CISOs
Develop an AI Security Strategy. Create a security strategy that addresses AI-specific risks and requirements. Define your target security state, assess gaps, and build an actionable roadmap. Align the strategy with both your enterprise security framework and AI adoption plans.
Build Security into AI Culture. Make security part of every AI project from day one. Connect security teams with AI developers and business units early. Clear communication between these groups and focus on collaboration helps catch issues before they become problems.
Show Security’s Business Value. Explain how security helps rather than hinders AI projects. Use examples that show how early security involvement speeds up deployment, protects company reputation, and builds customer trust. This helps get support and funding for security initiatives.
Strong security practices turn your team from project blockers into innovation enablers. This change helps organizations use AI effectively while managing risks.
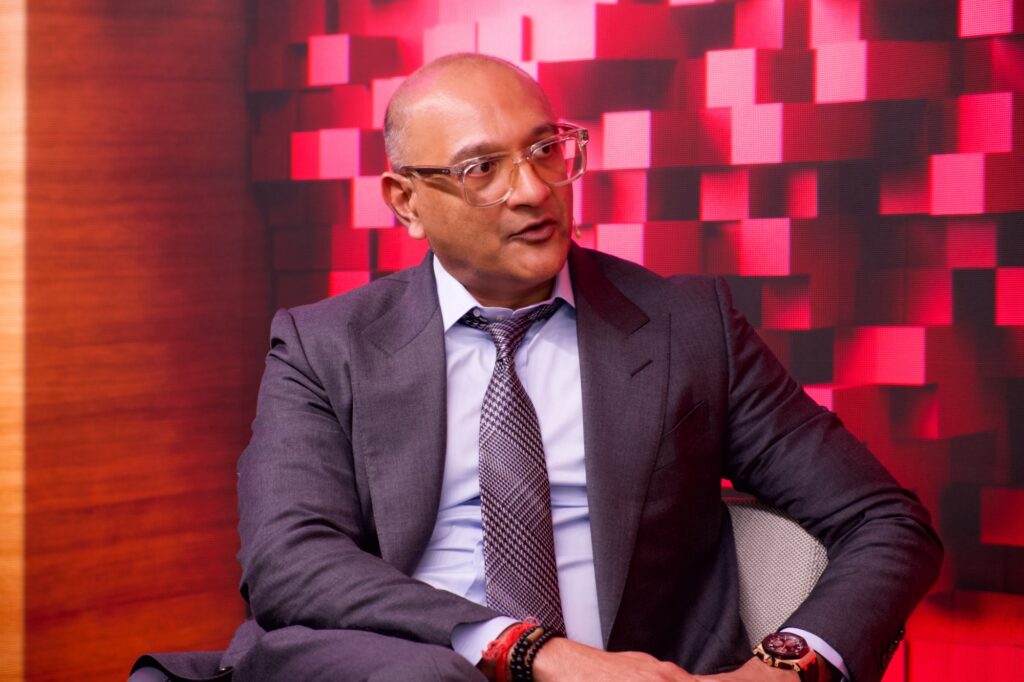
03: AI Supply Chain: Managing Third-Party Risks
Third-party AI tools, models and services add new security risks to organizations. Companies using external AI services can inherit security vulnerabilities and compliance problems. CISOs need clear strategies to handle these supply chain risks.
Key Insights from the Session
Third-Party AI is Common. Organizations regularly use external AI components, from open-source models to specialized services. Narvina Singh noted that most Credo AI customers use both internal and external AI systems. Companies rely on outside expertise to speed up AI development.
External Components Need Controls. Organizations struggle to govern AI systems that use third-party elements. These components must meet the same security standards as internal systems. Companies need ways to verify the security of AI tools they do not fully control.
Cyber Implications
Supply Chain Vulnerabilities. External AI components can introduce security weaknesses into your systems. These vulnerabilities affect your overall security posture and create new attack vectors. A recent World Economic Forum study found that 54% of large organizations cite third-party risk management as a major challenge in AI security.
Compliance Risk. Third-party AI services may not meet your regulatory obligations. This creates compliance gaps when external providers’ security controls don’t match your requirements. According to WEF research, 48% of CISOs report that ensuring third-party compliance is their main challenge in implementing cyber regulations.
Limited Visibility. Organizations often cannot fully examine third-party AI models and systems. This lack of transparency makes it harder to assess security risks and validate AI decisions.
Data Protection Challenges. Sharing data with external AI providers expands your data security perimeter. This requires additional controls to protect sensitive information across the AI supply chain. WEF reports that 69% of organizations struggle with complex regulations and verifying third-party compliance.
Key Recommendations for CISOs
Include Supply Chain in AI Security Framework. Ensure your security framework addresses unique AI supply chain risks. Include controls for managing third-party risks covering the entire lifecycle from procurement to deployment and beyond. Include provisions for operational activities like model updates, retraining, and secure decommissioning.
Prioritize Transparency and Explainability. Select AI vendors who provide visibility into their models, algorithms, and data sources. While complete explainability is not always possible, ensure vendors can explain their AI systems’ operation, training data, and potential biases.
Set Strong Security Requirements in Contracts. Include specific security requirements in AI vendor contracts. Beyond standard security clauses, define requirements for data usage in training, model testing, and validation. Add requirements for prompt injection, output filtering, and content monitoring if justified for your use case. Specify standards for model updates, retraining, and performance tracking. Include terms for incident response and liability for AI-specific incidents. Require documentation of training data, testing, and model explainability. Address regulatory compliance, user privacy in model interactions, and system decommissioning. Set clear terms for third-party model usage.
Adjust Vendor Risk Assessment. Adapt your vendor risk assessment process for AI providers. Beyond standard security controls, evaluate their AI development practices, training data security, and model governance. Check how they handle model updates, testing, and incident response. Assess their capabilities in bias detection, prompt injection prevention, and output filtering. Review their model documentation, explainability, and monitoring practices. Examine their use of third-party and open-source models. Verify their compliance with AI regulations and governance standards.
Monitor Third-Party AI Systems. Monitor the performance, security and compliance of third-party AI components regularly. Track vulnerability disclosures, security updates, changes in the vendor security posture, and model behavior issues that could indicate potential issues.
AI supply chain security requires careful attention to prevent vulnerabilities and compliance issues. Strong vendor assessment, continuous monitoring, and clear contract requirements help organizations use external AI securely. Focus on AI-specific risks while maintaining standard security practices.
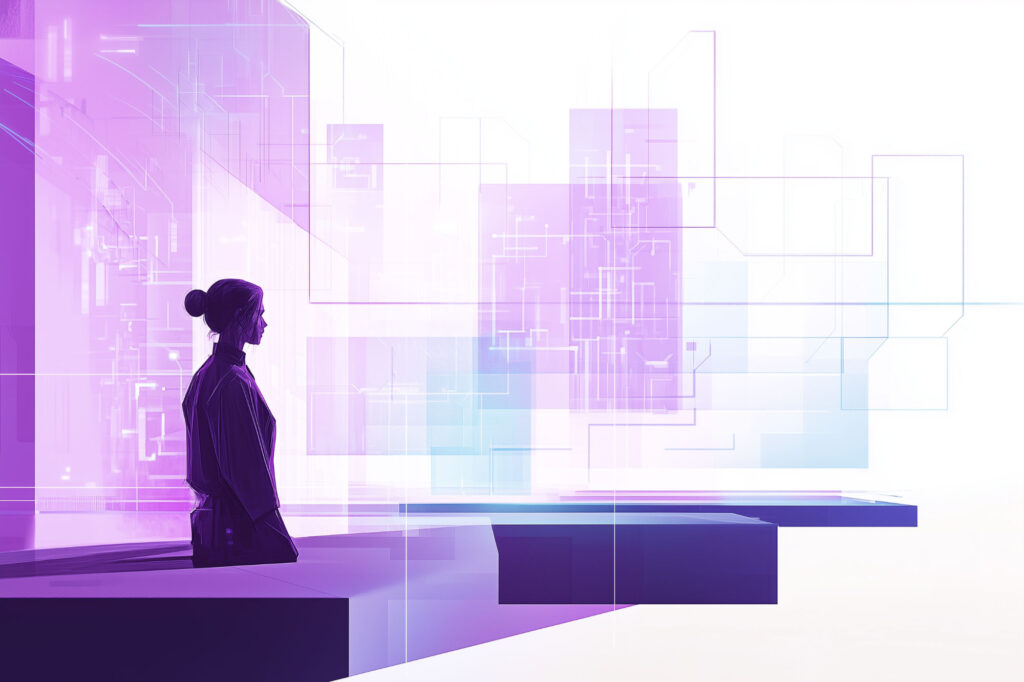
04: The Human Factor: AI Literacy and Skill Gaps
Organizations need employees who understand how to use AI systems securely. The Davos panel highlighted a critical gap in AI knowledge across organizations. This gap affects how quickly and safely companies can adopt AI.
Key Insights from the Session
Low AI Literacy. Narvina Singh pointed out that companies invest too little in AI education for employees, boards and executives. It can lead to issues when people do not understand AI capabilities, limitations and potential risks.
Shadow AI Usage. Employees often adopt AI tools without proper guidance or security oversight. The panel noted that this bottom-up adoption increases security risks.
Evolving Roles. Singh described how data scientists and policy managers are moving into AI governance positions. Organizations are also beginning to discuss new roles like “digital agent managers” for AI oversight.
Cyber Implications
Employee Security Risks. Staff who do not understand AI may be more susceptible to social engineering or other threats that exploit AI capabilities. They may also accidentally expose sensitive data or misconfigure AI systems, creating security gaps. According to Deloitte, 35% of organizations cite mistakes with real-world consequences as their top barrier to AI adoption. The WEF’s Global Cybersecurity Outlook 2025 reports that 55% of CISOs consider deepfakes a moderate-to-significant cyber threat to their organization.
Policy Compliance Issues. Security policies fail when employees do not understand the rationale behind them or how to follow AI security rules.
Security Talent Gap. Organizations struggle to find security professionals who can protect AI systems. The speed of AI adoption has created a shortage of qualified AI security experts. Deloitte reports that 26% of organizations see a lack of technical talent and skills as a key barrier to developing and deploying generative AI.
Key Recommendations for CISOs
Set AI Security Skill Requirements. Define the security knowledge needed for different AI roles. Use this to identify gaps and plan training. Include both technical and governance skills for AI development and operations teams.
Launch AI Security Training. Create training programs on AI security. Include practical guidance on protecting sensitive data in AI interactions, reviewing AI outputs for data leaks, identifying deepfakes and following AI security policies. Focus on safe practices when sharing data with AI systems.
Develop AI Security Expertise. Help your security team build AI security skills. Support them with specialized training, certifications, and hands-on experience with AI systems.
Build AI Security Teams. Hire specialists with AI security expertise. Work with universities and research groups to find talent. Ensure compensation matches the specialized skills required.
Support Ongoing Learning. Keep your team current on AI security developments. Enable them to attend key conferences and join professional communities. Share AI security knowledge across teams.
Strong AI security requires both technical expertise and security awareness across your organization. Focus on building these skills to protect your AI systems effectively.
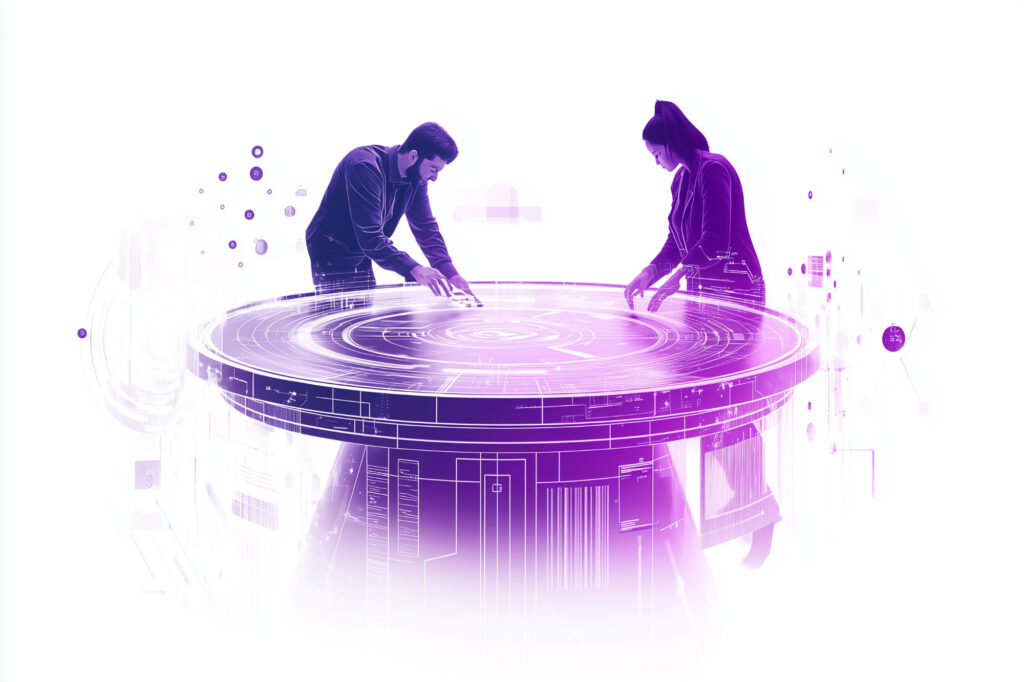
05: Agentic AI and the Future of Security
AI is evolving toward autonomous systems that can reason, plan and act independently. This shift brings new security challenges that CISOs must address.
Key Insights from the Session
AI Agents Coming. Arvind Jain described how AI agents will automate many knowledge work tasks. These agents will proactively support employees by anticipating their needs and assisting with their daily work.
Proactive AI Agents. Jain described a future where AI agents are proactive assistants. For instance, an agent might prepare a personalized “podcast-style” briefing for an employee’s commute based on their schedule and priorities.
Cyber Implications
New Attack Vectors. Agentic AI creates security risks beyond traditional threats. Attackers could manipulate agents by exploiting their decision-making processes, inject false data, or compromise agent operations.
Autonomous System Security. Securing AI systems that make independent decisions without direct human oversight requires new approaches. Standard security controls may not address agent-specific risks.
Agent Monitoring. Monitoring autonomous AI operations needs specialized tools and techniques. They must be able to track agent behavior, detect anomalies, and intervene when necessary.
Insider Threats. AI agents with access to sensitive data and systems could pose an insider threat if compromised or manipulated. This requires strict access control and continuous activity monitoring.
Key Recommendations for CISOs
Update AI Security Strategy. Adapt AI security strategy and framework for autonomous AI. Add agent-specific controls, such as monitoring, behavior analysis, and authority limits.
Study Agent Security. Research methods to secure autonomous systems. Focus on agent behavior monitoring, threat modeling, and secure agent-to-agent communication. Collaborate with industry groups, academic institutions or research labs specializing in AI security.
Maintain Human Control. Design AI workflows with human oversight. Set clear limits on autonomous operations and enable manual intervention when needed.
Ensure Agent Visibility. Implement controls to track how AI agents operate and make decisions. Monitor agent actions and identify potential security issues early.
CISOs must prepare for AI agents becoming part of the workforce. Focus on understanding agent-specific risks and developing appropriate security controls.
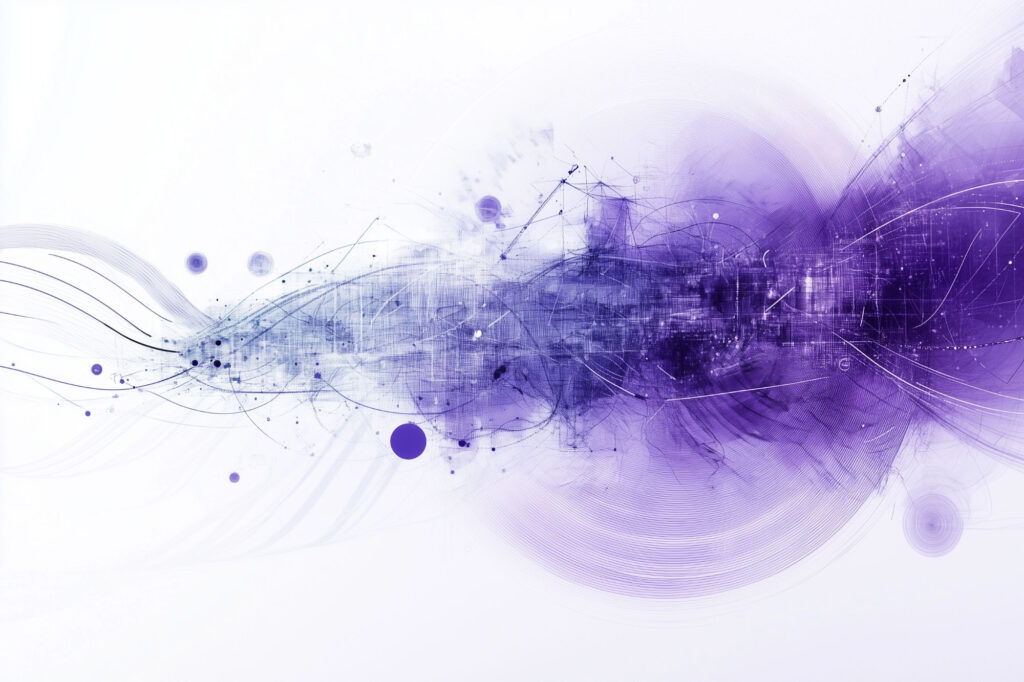
06: Conclusion
The AI House Davos panel made clear that AI is now central to business operations.
CISOs face immediate challenges from rapid AI adoption, autonomous AI systems, and complex AI supply chains. Their task is to enable secure AI use while protecting against new risks.
Security teams must help organizations use AI safely. This means building AI security awareness, addressing AI-specific threats, and implementing effective controls. As AI becomes essential to work, CISOs play a crucial role in securing its use.